Metadata’s Journalistic Moment
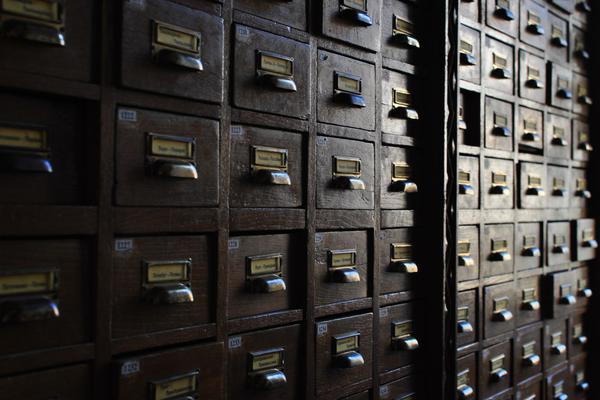
In a world awash in news, the application of metadata – bits of digital information that describe the content to which it is attached – is an essential if oft-loathed step in the journalism process. But with automation, precise keyword tagging is helping news organisation’s rethink how their content is delivered to their audiences.
For Carl-Gustav Lindén, an Associate Professor of Data Journalism at the University of Bergen, this revelation has the potential to strengthen journalism’s fractured business model. We recently spoke with Lindén to understand why a long under-appreciated part of the news industry is finally getting its due.
To many journalists, and even journalism scholars, metadata is a dry topic. But this is changing. What’s going on?
Indexing a diverse set of knowledge has been a core idea for newsrooms ever since they started publishing. In the past it was for archival purposes. But newspapers have recently realized that they must have good metadata to operate in the digital world and to monetize their product.
Unfortunately, this knowledge alone is not enough. One problem is that journalists don't think that tagging content [the process of manually creating metadata] is something they should do. Perhaps interestingly, neither do I. Why? Because today we have automated systems for that. When journalists tag content manually they usually do it wrong.
Some legacy media like The New York Times disagree; they use a semi-automated model, where their journalists are adding static metadata but also getting [tagging] suggestions from the systems. They also have taxonomists literally walking around the newsroom to help journalists with the tagging.
So The New York Times, one of the world's leading innovators in storytelling and news technologies, is still using people in the process of keyword tagging – even though we have great software to do it automatically?
Yes, exactly. They have a team of three taxonomists who walk around giving advice on what to do and how to tag stories correctly. The last thing I heard a few weeks ago from within the newsroom is that they will keep this system of semi-automated tagging because it's working well so far for them. Machine learning and keyword extraction tools do work quite well, too, but maybe not well enough for The New York Times.
What about smaller publishers? Are they able to use automation opportunities?
One of the problems with news automation and AI is that some publishers believe its best use case is generating news texts. But so-called “bulk news” churned out by AI is of little commercial value. We already suffer from the overproduction of news. Therefore, to use AI well we need new ideas.
The second challenge is the scope of the dataset used to train the metadata-tagging algorithms. Large organisations have huge archives for machine learning purposes – whether its millions of photos or billions of lines of text. Small publishers don’t have that source data for training automation tools or natural language generation systems.
A third challenge is creativity. During my research I realised that most AI is template based, meaning, journalists are writing text templates to be used by computers to churn out a story. This isn’t AI. However, studying what Nordic newsrooms are doing with metadata, I found that this is the foundation for more advanced forms of news automation.
For instance, in 2018, I read about a German company creating some 8,000 templates for the Bundesliga. I was excited because I thought there was actual machine learning involved. But when I contacted them, they said, ‘No, we just spent three months writing the templates. It's all made by hand.’ So, that shows just how little progress there is in AI-generated news. And it’s why, in my view, the automated generation of metadata has far more potential.
What are some of the most interesting use cases that you've seen regarding metadata in newsrooms?
In the Nordic countries, news organisations are creating advanced data management systems that make it possible for subscribers to automatically receive stories that are relevant to them. For instance, let’s say there is someone from Tromsø, in northern Norway, who is active in politics on the European level. The local newspaper in that part of Norway might have no idea about this person’s activity in Brussels. By tagging these stories with the correct metadata, subscribers can automatically be informed of content that is of interest.
Have we reached an inflection point in the industry with regards to recognition of metadata’s importance?
It's the absolute driver of journalism as a digital business model. Gone are the days of journalists refusing to tag their stories; failure to do so essentially means no one will find and read what you’ve written.
But even then, it all comes back to quality content. For instance, if we look at NTB in Norway or Bonnier News in Sweden, they’ve been quite successful in reinventing their businesses. And it's all based on quality journalism. It's all about having a clear and crisp value proposition – tell customers that it is journalism that we do, and it is journalism that customers pay for. People get that message. I think that's been a problem with the media – having a really good value proposition. For too long it's been a mixed message towards advertisers and subscribers.
Many AI and metadata automation tools have been created for a Western, and largely American, context. What are some of the challenges that this creates for news organisations elsewhere?
To give the right labels to things it’s important to have the social context supporting them. For instance, consider the education system in Norway. In the US there is one way of thinking about how education works – with format, structure, curriculum – and that is not easily transferable to the Nordic reality. So, you need to adapt the metadata. The same goes for any political system. The political systems of the US and the UK aren't in any way comparable to the Nordic system. Again, the metadata needs to be adapted to the content.